Scientists can now tell who is about to fall ill, they claim
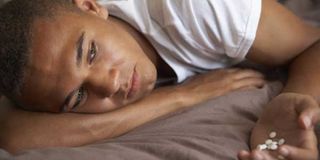
By combining brain imaging data and supercomputers to identify patterns in neuroimaging data, researchers in the United States could predict the risk for mental disorders such as depression or dementia. PHOTO | FILE
What you need to know:
- But identifying the tell-tale signs is not as simple. Mr Schnyer used a Stampede supercomputer to train a machine-learning algorithm that can identify cohesions among hundreds of patients using magnetic resonance imaging (MRI) brain scans and other relevant factors to provide accurate predictions of risk for those with depression and anxiety.
- According to the study, the team used a machine learning approach to classify individuals with major depressive disorder with roughly 75 per cent accuracy.
It may be possible to detect who might be vulnerable to a mental illness before its onset using brain imaging, neuroscientists have said.
By combining brain imaging data and supercomputers to identify patterns in neuroimaging data, researchers in the United States could predict the risk for mental disorders such as depression or dementia.
Scientists have long studied mental disorders by examining the relationship between brain function and structure in brain imaging data. “One difficulty with that work is that it is primarily descriptive,” said David Schnyer, a cognitive neuroscientist and professor of psychology at the University of Texas.
“The brain networks may appear to differ between two groups, but it does not tell us about what patterns actually predict which group you will fall into.”
MAPPING DEPRESSION
But identifying the tell-tale signs is not as simple. Mr Schnyer used a Stampede supercomputer to train a machine-learning algorithm that can identify cohesions among hundreds of patients using magnetic resonance imaging (MRI) brain scans and other relevant factors to provide accurate predictions of risk for those with depression and anxiety.
“We are looking for diagnostic measures that are predictive for outcomes like vulnerability to depression or dementia,” he said.
The team of researchers drawn from four US universities published their analysis of a proof-of-concept study in the scientific journal Elsevier. According to the study, the team used a machine learning approach to classify individuals with major depressive disorder with roughly 75 per cent accuracy.
Machine learning is a subfield of computer science that involves the construction of algorithms that can “learn” by building a model from sample data inputs and then make independent predictions on new data.
For their study, involving analysis of brain data from 52 treatment-seeking participants with depression, and 45 healthy control participants, Mr Schnyer used a type of machine learning called Support Vector Machine Learning.
The researchers provided a set of training examples, each marked as belonging to either healthy individuals or those who have been diagnosed with depression. To compare the two, a subset of depressed participants was matched with healthy individuals based on age and gender, bringing the sample size to 50.
“Not only are we learning that we can classify depressed-versus-non-depressed people using DTI data, we are also learning something about how depression is represented within the brain,” said Christopher Beevers, a professor of psychology and director of the Institute for Mental Health Research at the University of Texas, Austin.